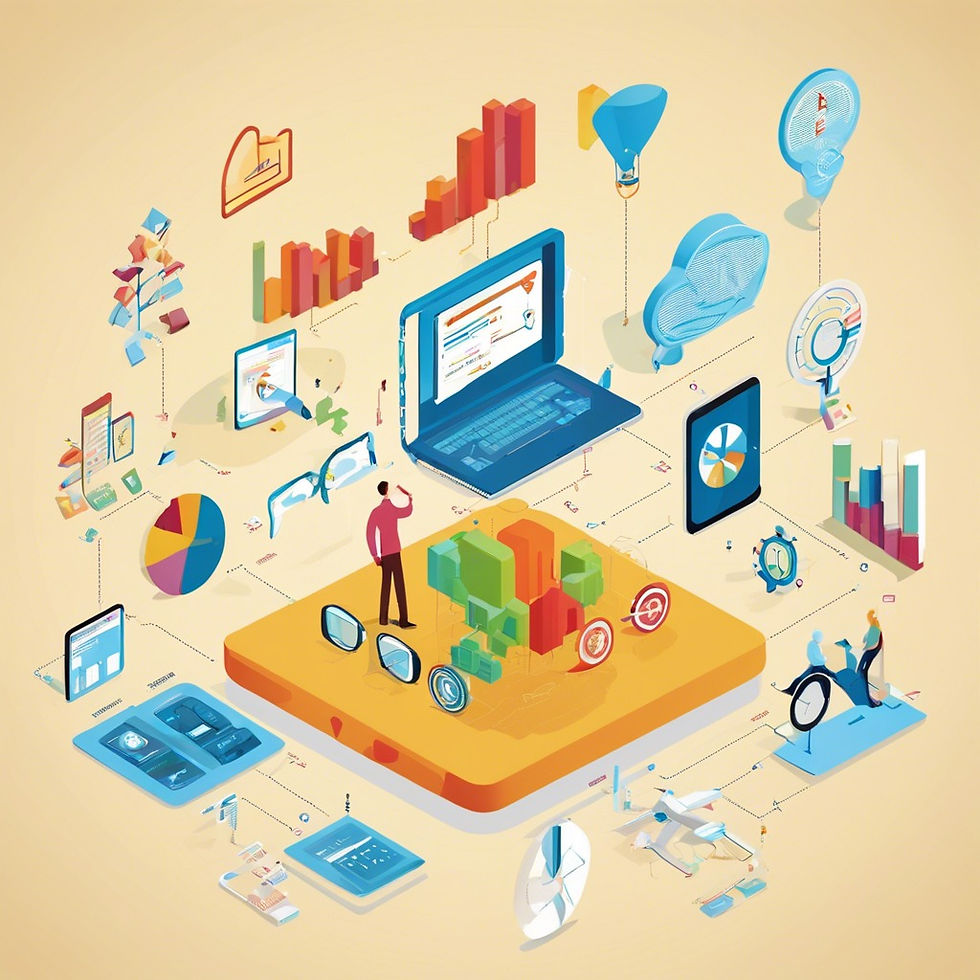
In an era defined by data abundance and technological advancement, businesses and organizations are increasingly turning to predictive analytics to gain insights, make informed decisions, and stay ahead of the curve. Predictive analytics, a branch of data analytics, leverages historical data, statistical algorithms, and machine learning techniques to forecast future trends, behaviors, and outcomes with a high degree of accuracy.
At its core, predictive analytics is about using data to anticipate what is likely to happen in the future. By analyzing patterns, trends, and relationships within data sets, predictive analytics enables organizations to identify hidden insights and make proactive decisions that drive business growth and success. From predicting customer behavior and optimizing operations to mitigating risks and seizing opportunities, the applications of predictive analytics are vast and diverse.
One of the most common applications of predictive analytics is in the realm of sales and marketing. By analyzing customer data, such as purchase history, browsing behavior, and demographic information, businesses can develop predictive models to forecast future sales, identify high-value customers, and personalize marketing campaigns. This targeted approach not only increases customer engagement but also enhances the overall effectiveness of marketing efforts.
In the field of finance, predictive analytics plays a crucial role in risk management and fraud detection. Banks and financial institutions use predictive models to assess creditworthiness, predict loan defaults, and detect fraudulent activities. By analyzing historical transaction data and identifying anomalies or suspicious patterns, organizations can mitigate risks, minimize losses, and safeguard against financial fraud.
Predictive analytics also holds significant promise in healthcare, where it can be used to improve patient outcomes and optimize resource allocation. By analyzing electronic health records, medical histories, and diagnostic data, healthcare providers can develop predictive models to anticipate disease progression, identify high-risk patients, and optimize treatment plans. This proactive approach not only improves patient care but also reduces healthcare costs and enhances overall efficiency.
In addition to its practical applications, predictive analytics is also driving innovation and transformation across industries. In manufacturing, predictive maintenance enables organizations to predict equipment failures before they occur, thereby minimizing downtime, reducing maintenance costs, and optimizing production processes. In transportation and logistics, predictive analytics is used to optimize route planning, fleet management, and inventory forecasting, leading to improved efficiency and cost savings.
Despite its numerous benefits, implementing predictive analytics can pose challenges for organizations, including data quality issues, resource constraints, and privacy concerns. Building robust predictive models requires access to high-quality data, sophisticated algorithms, and skilled data scientists. Furthermore, organizations must navigate ethical considerations and data privacy regulations to ensure that predictive analytics initiatives adhere to legal and ethical standards.
As we look to the future, the role of predictive analytics will only continue to grow in importance. With advances in artificial intelligence, machine learning, and big data technologies, predictive analytics holds the potential to revolutionize decision-making processes, drive innovation, and create value for organizations across sectors. By harnessing the power of predictive analytics, businesses can unlock new opportunities, mitigate risks, and chart a course towards success in an increasingly complex and dynamic world.
Comments